Data Governance for the Tax Administration. A CIAT publication
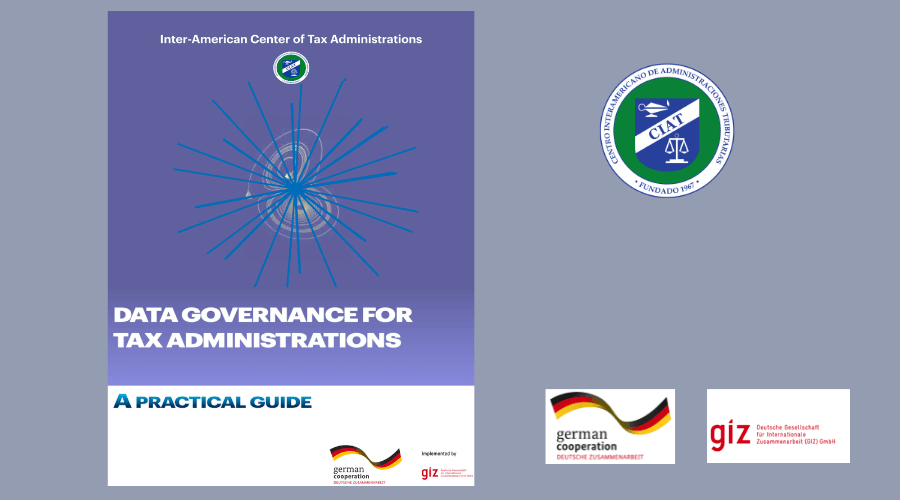
At the end of the 80s, and for a few more years, the need to specialize technology people in large organizations, such as tax administrations, was evident. Large data centers required computer operators to run jobs written in JCL, those who knew about CICS, those of terminal controllers, and those who dealt with data. In some cases, relational database handlers were starting to be used and, of course, SQL specialists came with them.
On one occasion, a particular request came to the computer center. A statistic was required based on comparing the tax returns of the last period with those of the current period, and seeing the total variation of gross income for some taxpayers of a certain region and economic activity. A willing newcomer who mastered SQL and had participated in the migration of data to the relational database put together the query in a very short time, he ran it and sent to print the result, proudly delivering it very quickly. All in less than five minutes.
One floor above, the person responsible for transferring the information to the minister’s office received the sheet with the data. When he saw the result, he was surprised for two reasons, the first, because something that usually took a couple of days to execute was completed in a few minutes, a huge increase in efficiency. The second reason is that he found out that the GDP of an economic sector had tripled in a year without significant inflation. The head of the department went down to the computer center and looked for the newly arrived professional. He scolded him. “How can you deliver this without validating?” he said to her in a high-pitched tone of voice. “The query is correct” the developer replied bravely. “I can assure you, what is wrong is the data.” And it was true.
With the same skill as before, the developer searched for the most valuable of the selected tax returns. The problem was coming from a single return, where an income field had the taxpayer’s number. Very quickly, basically by adding one more line in the part of the where, a result was obtained that now looked reasonable. A girl who had quietly witnessed the whole incident asked earnestly: “Is that mistake going to have been incorporated in the transcription center, or would it come from the taxpayer?” and she continued “How do we know there are no more mistakes?”. No one answered. They did not know.
For purely statistical purposes, removing that tax return from the sample was certainly sufficient, but in a scenario where data is used to make specific decisions, data quality issues do not focus exclusively on identifying the big errors that can distort a statistic, they are about decisions with individual consequences. In addition, it becomes more important when the making of those decisions incorporates artificial intelligence processes such as, for example, machine learning, which are fully supported on the available data.
The need to take care of data quality today is more complex. Visually verifying whether an affidavit had a source error or was incorporated by the two transcribers which captured and validated the capture process may have been reasonable 40 years ago, but visually validating the thousands of electronic documents that arrive at an administration in a minute is not possible To that we must add the concerns about privacy, the ethical treatment of data, the demand for greater transparency, the exchange of information, the right to be forgotten, the certainty that the data will not be altered or destroyed accidentally or deliberately. All this analysis extends over structured data, of course, but it extends to unstructured data, for example, audio or video recordings about the officials’ interactions with the taxpayers.
Tax administrations, like any organization that makes intensive use of data, execute a governance over these processes and over the data they have or want to have and their life cycle, but this management is not necessarily formalized or sufficiently mature. The gradual absorption of huge amounts of data from different sources increases the problem. It is worth asking, is it always possible to identify, for example, who is responsible for the quality of a particular data domain, is it someone from the technology area, or from the user areas, and in that case from which, and how. As an exercise, ask yourself who is responsible for ensuring that the data quality of the taxpayers’ phone number is reasonable. Surely there are validations immersed in the data capture at the time of registering the taxpayer, and that guarantees that a phone number looks like a phone number, but it says nothing about whether that phone number really belonged to the taxpayer at the time of registration, but much less if it is still valid a few years later. In addition, it is also difficult to determine if someone in the organization knows what percentage of phone numbers may be invalid to continue considering that information as useful, and how it is verified, and who and when can consult it, or if it can be shared with other organizations, or published on a website, or decide to discard it, if it is no longer useful.
Today, several data-intensive organizations are concerned with establishing, formalizing, improving and maturing the governance of their data and, in addition, expanding employees’ abilities to understand, share knowledge and have meaningful conversations about data. There are techniques, methodologies, maturity models and a lot of literature on this. Some tax administrations have initiated or are considering initiating this process. However, doubts arise about what and how to do it, or where to start, but also about how to develop these practices without necessarily establishing too rigid and bureaucratic mechanisms.
That is precisely why we have prepared this guide on data governance that we are making available to you. This effort had the support of the GIZ and seeks to answer some of these questions: what practices and competencies should be created? what governance structures should be implemented? how to evaluate the maturity we have and the one we want to achieve? what path or roadmap can we take? how to start with a simplified mechanism that does not spread in a bureaucratic way?
This publication can be downloaded in English from the CIAT library
Greetings and good luck.
1,588 total views, 1 views today