Explainable Artificial Intelligence (XAI)** and its importance in Tax Administration
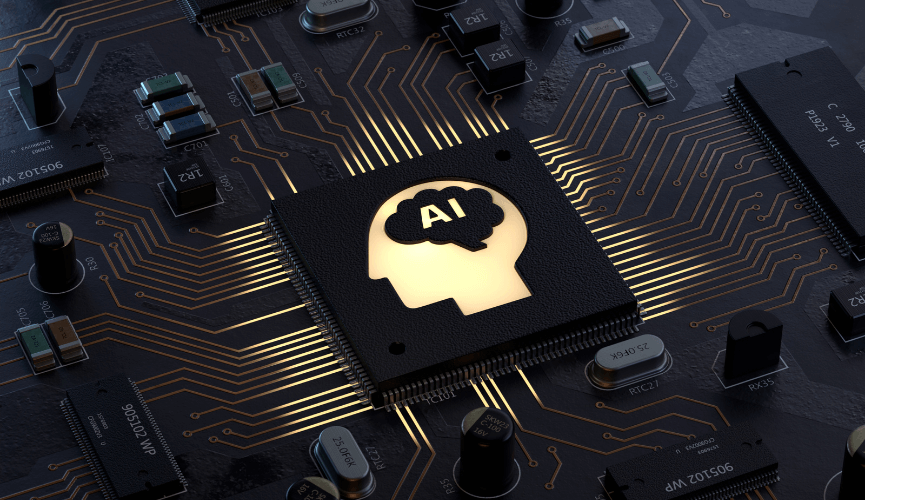
General aspects
Artificial intelligence (AI) has been impacting not only business, but also our daily lives, from facial and image recognition to predictive analytics based on machine learning, conversational applications, autonomous machines and hyper-personalized systems[1]. The kinds of decisions and predictions that AI-enabled systems make are becoming much deeper and they may become critical to life, death, and personal well-being (Schmelzer, 2019).
Although they affect everyone’s life, few of us are able to understand how and why AI systems make their decisions and how they apply in our lives. Nor is it possible to reconstruct the path followed by many algorithms based on the results found. This opacity leads to mistrust in the results. Particularly noteworthy are applications in healthcare, driverless cars and drone operations in warfare. Today, it is increasingly important, for certain applications, to also recognize the fundamental human rights and legal impacts associated with AI system decisions or recommendations. Furthermore, in cases brought to court, judges increasingly confront machine learning algorithms, seeking explanations on which they can base their decisions.
This context strengthens the debate about which decisions based on AI algorithms need explanation, and with what types of clarifications. Thus, the explanation of how they have reached a certain conclusion could be important to allow those affected by it, to challenge or change that result.
The AI algorithms
AI is based on distinct types of algorithms, which act on data sets, in models such as machine learning (ML), deep learning (DL) and neural networks (NN). According to IBM, too many of these models look like “black boxes”, which are impossible to interpret. Neural networks, used in deep learning, are often the most difficult for humans to understand. Bias, often based on race, gender, age, or location, is a risk that has always been present in AI model training. In addition, according to the same source, the performance of the model may vary or degrade, as the production data is different from the training data. This adds an additional decisive argument for an institution to continuously monitor and manage AI models, promote elucidation and evaluate the decisions.
Algorithms and legal consequences
Operational and legal problems with algorithm-based systems are not new, the literature has some examples, such as the case of the fraud detection system in unemployment insurance claims of the state of Michigan (United States), which improperly charged fines and blocked returns of taxes to thousands of beneficiaries, and now responds to legal processes. In 2019, a Dutch court determined that an algorithm used in the detection of social assistance fraud violated human rights and ordered the government not to use it anymore (Wykstra, 2020).
The tax administration, which is pleased with the potential of AI algorithms to improve its performance in different areas, must also consider the effects of algorithms when the issue is subject to discussion in court.
Kuzniacki and Tylinski (2020) indicate that the greatest source of risks for the integration of AI into the legal process in Europe are the General Data Protection Regulations-RGPD[2] and the European Convention on Human Rights-ECHR[3]. The legal articles mentioned by the authors are the following:
Kroll, Huey et al. (2017) indicates that the use of advanced and complicated AI models, such as neural networks, is not yet transparent enough to be the only tool that makes tax decisions without human supervision. Therefore, instead of neural networks, they recommend in these cases to use easily explainable AI models, such as decision trees, different versions of the simultaneous use of multiple machine learning methods (ensemble learning), so that it is possible to provide meaningful information about the logic involved in the model. The results must appear in an intelligible way by humans, as well as the factors that led the model to make the decision to apply or not apply the tax law. This allows people to challenge such decisions, even before they are officially issued.
What is XAI?
The context described above led to the need for more advanced and structured studies on the subject, consolidated in a new field, named Explainable Artificial Intelligence or XAI.
We can define XAI as a set of processes and methods, which allows people to understand and trust the results and guidance generated by machine learning algorithms. The Explainable AI can describe an AI model, its expected impact, and potential biases[4]. Anyway, it promotes accountability (algorithm accountability).
In this context, XAI also helps promote end-user trust, model auditability, and productive use of AI, as well as mitigating reputational, security, and legal compliance risks.
Companies such as IBM have identified the importance of XAI and offer resources such as blogs and toolkits, so their customers may start studying this field.
It seems that the legal area will be the main interested party and the functional driver of the XAI studies of a tax administration.
In the current situation, in European countries and in the United States, there is no clear guidance from the courts in cases dealing with decisions based on algorithms. Since there are no consolidated precedents, courts may take decisions based on the criteria of each judge.
Deeks (2021) argues that judges will face a variety of cases in which they must demand explanations for algorithmic decisions, recommendations or predictions. Due to these demands, the judges will play a fundamental role in shaping the nature and form of XAI. Using the tools of common law, courts can construct what the role of the XAI should be in different legal contexts, including criminal, administrative and civil cases. Thus, he concludes that the judges are the main actors in the definition and meaning of XAI.
The same author affirms that as the courts employ and develop the existing case law in the face of predictive algorithms, which arise in a series of litigation, they will create the “common law of XAI”, a law sensitive to the requirements of different audiences (judges, jurors, plaintiffs or defendants) and different uses for the provided explanations (criminal, civil or administrative law settings).
Final remarks
The need to explain AI algorithms is still a new topic for tax administrations in LAC (and in the world). But the progress made in the use of AI makes it available in all areas of taxation, and lawsuits will surely follow, as the legal principles and general data protection laws in force or in preparation are often based on principles of their European and North American models.
According to Doshi-Velez, Kortz et al. (2017), in this new field of legal and technological research, the role of AI algorithm explanation to guarantee its responsible use must be periodically reassessed to adapt to a changing technological world. Access to greater computational resources can reduce the computational burden associated with explanation, but it also allows the use of even more sophisticated algorithms, increasing the challenges associated with an accurate explanation.
On the other hand, not every AI solution needs to be “explainable.” Thus, the first step is to identify this need before the design.
Likewise, it is well-advised to be alert for the oncoming controversies.
Bibliographic References:
Deeks, A. 2021. The Judicial demand for explainable Artificial Intelligence. Columbia Law Review. Vol. 119. No. 7. Available at: https://www.columbialawreview.org/content/the-judicial-demand-for-explainable-artificial-intelligence/
Doshi-Velez, F., Kortz, M. et al. 2017. Accountability of AI under the law: The role of explanation. Working Draft from Berkman Klein Center Working Group on AI Interpretability. Available at: https://arxiv.org/ftp/arxiv/papers/1711/1711.01134.pdf
Kroll, J., Huey, J. et al. 2017. Accountable algorithms. University of Pennsylvania Law Review. Available at: https://scholarship.law.upenn.edu/penn_law_review/vol165/iss3/3/
Kuzniacki, B., Tylinski, K. 2020. Legal risks stemming from the integration of artificial intelligence (AI) to tax law. Kluwer International tax blog. Available at: kluwertaxblog.com/2020/09/04/legal-risks-stemming-from-the-integration-of-artificial-intelligence-ai-to-tax-law/
Schmelzer, R. 2019. Understanding Explainable AI. Available at: https://www.forbes.com/sites/cognitiveworld/2019/07/23/understanding-explainable-ai/
Wykstra, S. 2020. How the government’s use of algorithm serves up false fraud charges. Salon.com. Available at: https://www.salon.com/2020/06/14/how-the-governments-use-of-algorithm-serves-up-false-fraud-charges_partner/
**Explainable AI in English
[1]Hyper-personalization is the most advanced way that brands can tailor their marketing to individual customers. It creates personalized and specific experiences using data, analytics, artificial intelligence and automation (Deloitte).
[2]See https://gdpr-info.eu/
[3]See https://www.echr.coe.int/Documents/Convention_ENG.pdf
[4]See https://www.ibm.com/watson/explainable-ai
2,507 total views, 1 views today